Unlock the Power of Machine Learning with Image Annotation Tools
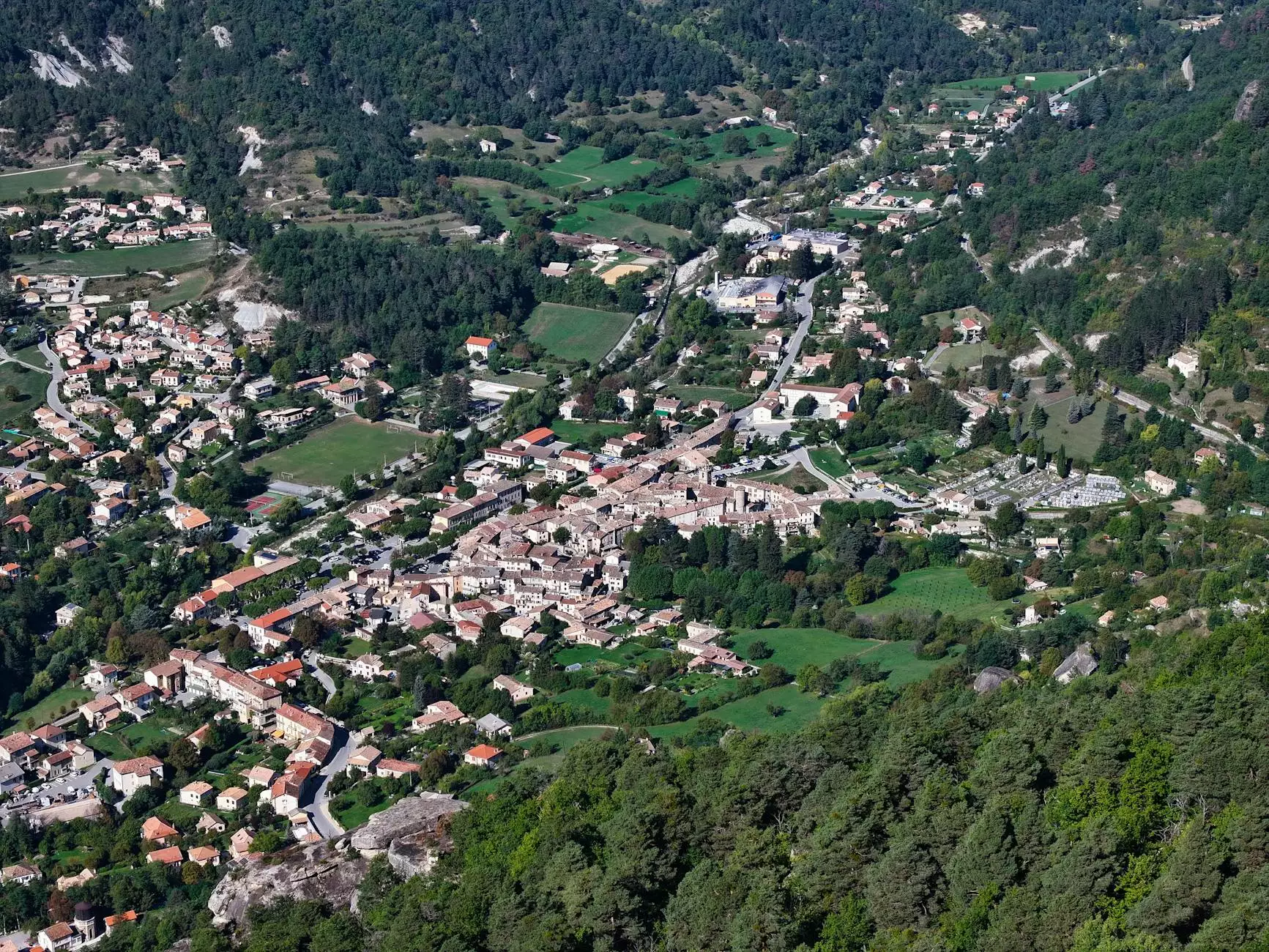
In today's data-driven world, image annotation tools for machine learning have become integral to the success of artificial intelligence applications. These resources provide the necessary infrastructure to prepare visual data for machine learning algorithms, enabling computers to understand and interpret images just like humans do. This article dives deep into the myriad ways data annotation tools serve not just the field of AI but also the industries leveraging this technology.
What is Image Annotation?
Image annotation involves labeling images with appropriate tags and descriptions to create a structured dataset. This process allows machine learning models to recognize patterns and features from the images, ultimately improving their accuracy and efficiency.
Why Are Image Annotation Tools Essential for Machine Learning?
The role of image annotation tools in machine learning cannot be overstated. Here’s why they are crucial:
- Enhancing Model Accuracy: Annotated datasets help in training models to make accurate predictions.
- Streamlining the Learning Process: Annotations provide contextual understanding for algorithms, speeding up their learning time.
- Facilitating Quality Control: Well-annotated images ensure consistent quality in data that enhances model reliability.
- Guiding Automated Processes: Accurate annotations allow automation in various fields, from healthcare to manufacturing.
The Different Types of Image Annotation
Understanding the types of annotations is crucial for selecting the right tool for your project:
- Bounding Boxes: These are rectangular boxes drawn around objects in images to identify and locate them. Commonly used for object detection tasks.
- Semantic Segmentation: This method annotates every pixel of an image, providing pixel-wise classifications to identify objects or boundaries.
- Instance Segmentation: Similar to semantic segmentation, but it differentiates between separate instances of the same object.
- Landmark Annotation: This involves placing points on specific features of an object, such as facial keypoints for facial recognition.
- Text Annotation: Useful in scenarios involving text recognition within images, highlighting and categorizing text segments.
How to Choose the Right Image Annotation Tool
Choosing the appropriate image annotation tool for machine learning is essential for efficient workflow and quality results. Here are some factors to consider:
- User-Friendliness: Look for intuitive interfaces that make the annotation process smooth.
- Customization Options: Tools should allow you to customize annotation types according to your project needs.
- Scalability: Ensure the tool can handle projects of varying sizes, from small datasets to massive image collections.
- Integration Capabilities: Tools should integrate easily with your existing workflows and machine learning models.
- Support and Documentation: Comprehensive support and guides can significantly ease the learning curve.
Keylabs.ai: Your Partner in Image Annotation
At Keylabs.ai, we offer a comprehensive data annotation platform designed to empower businesses in their machine learning journeys. Our platform provides:
- Expert Annotations: Our team of data annotators ensures high-quality, accurate image annotations tailored to your specific requirements.
- Custom Workflow: We work with clients to establish workflows that align with their project goals and timelines.
- Advanced Tools: Leverage our suite of tools that support various annotation types, enhancing efficiency and accuracy.
- Scalable Solutions: Whether you need to annotate thousands of images or just a few, our platform adapts to your needs.
- Reliable Support: Our dedicated support team is ready to assist you throughout the process to overcome any challenges.
The Impact of Image Annotation on Various Industries
The implications of effective image annotation extend across numerous sectors, showcasing its versatility. Here are a few notable examples:
Healthcare
In healthcare, image annotation tools are instrumental in diagnosing diseases through medical imaging. Accurate annotations of X-rays, MRIs, and CT scans help in training models to detect anomalies, thus assisting radiologists in making informed decisions.
Autonomous Vehicles
Self-driving cars depend on image annotation for object detection and scene understanding. Annotations enable the models to identify pedestrians, traffic signals, and other vehicles, thereby reducing the likelihood of accidents.
Retail and E-commerce
In retail, image annotation enhances product recognition and classification in e-commerce platforms. Annotated images can improve search functionalities and provide personalized recommendations, leading to a better customer experience.
Agriculture
Farmers use annotated images for precision agriculture, employing drone imagery to monitor crop health. Annotation helps identify plant diseases, pest infestations, and optimal harvesting times, ultimately improving yield.
Security and Surveillance
Security systems utilize image annotation for identifying suspicious behaviors and detecting unauthorized access. Machine learning models trained with accurately annotated surveillance footage can better predict and prevent security threats.
Best Practices for Image Annotation
For businesses looking to implement image annotation, adhering to best practices is crucial:
- Consistency is Key: Ensure that all annotations are consistent across datasets to avoid confusion during training.
- Utilize Guidelines: Create guidelines for annotators to standardize the annotation criteria, ensuring reliability and quality.
- Regular Review: Periodically review annotations to retain quality standards and make necessary adjustments as projects evolve.
- Consider Automation: Explore automated annotation solutions where applicable, especially for high-volume datasets, to save time and costs.
Future Trends in Image Annotation for Machine Learning
The landscape of image annotation tools for machine learning is continually evolving. Here are some future trends to watch for:
- Increased Use of AI: AI-powered annotation tools are expected to become more prevalent, reducing the need for manual input.
- Real-time Annotation: As demands for faster processing grow, real-time annotation capabilities will likely become a key feature.
- Collaborative Annotation Platforms: Tools that allow multiple users to work simultaneously on annotations may enhance workflow efficiency.
- Integration with AR/VR: As augmented and virtual reality become more integrated with data, the need for complex image annotations will increase.
Conclusion
As machine learning continues to advance, the role of image annotation tools becomes more prominent. Leveraging a robust data annotation platform like Keylabs.ai can significantly enhance the quality and efficacy of your datasets. Whether you are in healthcare, automotive, security, or any other industry, understanding and implementing effective image annotation strategies will set your projects up for success. Invest in the right tools and practices today to unlock the full potential of machine learning!